GenHMR: A Breakthrough in 3D Human Pose and Shape Estimation – Accepted at AAAI 2025
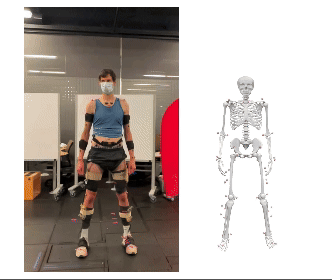
We are pleased to announce that our paper, “GenHMR: Generative Human Mesh Recovery for Accurate 3D Pose and Shape Estimation,” has been accepted for presentation at AAAI 2025. GenHMR introduces a novel generative framework that surpasses existing methods in parametric 3D human pose and shape (HPS) estimation, offering state-of-the-art accuracy and robustness.
Advancing 3D Human Mesh Recovery
Traditional deterministic and probabilistic methods for 3D pose estimation struggle with depth ambiguity, occlusions, and uncertainty in 2D-to-3D mappings. GenHMR redefines the problem by explicitly modeling uncertainties and leveraging generative modeling techniques to achieve high-fidelity 3D reconstructions.
Key Innovations of GenHMR:
- Generative HMR Reformulation: Moves beyond deterministic pipelines by modeling uncertainties in 2D-to-3D mapping, effectively addressing depth ambiguities and occlusions.
- Pose Tokenizer (VQ-VAE): Encodes 3D poses into discrete tokens, ensuring compact, kinematically valid representations for high-quality reconstructions.
- Image-Conditional Masked Transformer: Learns probabilistic pose distributions by predicting masked pose tokens, capturing complex joint dependencies for enhanced 3D accuracy.
- Uncertainty-Guided Sampling: Iteratively refines pose predictions, progressively improving accuracy by re-predicting low-confidence tokens during inference.
- 2D Pose-Guided Refinement: Ensures precise alignment between the reconstructed 3D mesh and 2D pose cues, enhancing final outputs.
Setting a New Standard for 3D Human Mesh Recovery
By combining generative modeling, iterative refinement, and uncertainty quantification, GenHMR outperforms existing methods in both accuracy and robustness, establishing a new benchmark for 3D human pose estimation.
Acknowledgments
This research was made possible through the contributions of Pu Wang, Ekkasit Pinyoanuntapong, Hongfei Xue, Srijan Das, and Chen Chen.
📄 Read the Full Paper: Link
🔗 Project Page: Link
Stay connected for more updates on advancements in human-centric AI and 3D vision research.